Big Data in Manufacturing: Using Analytics in Production
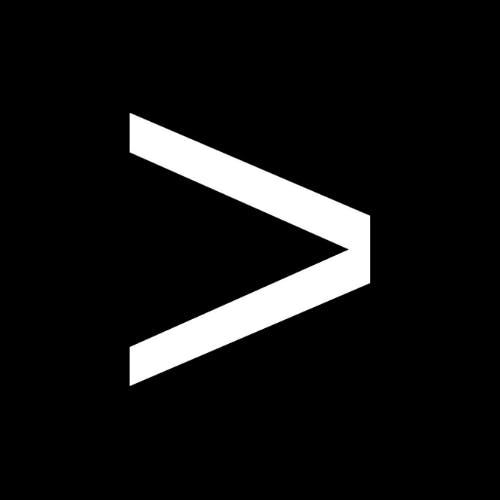
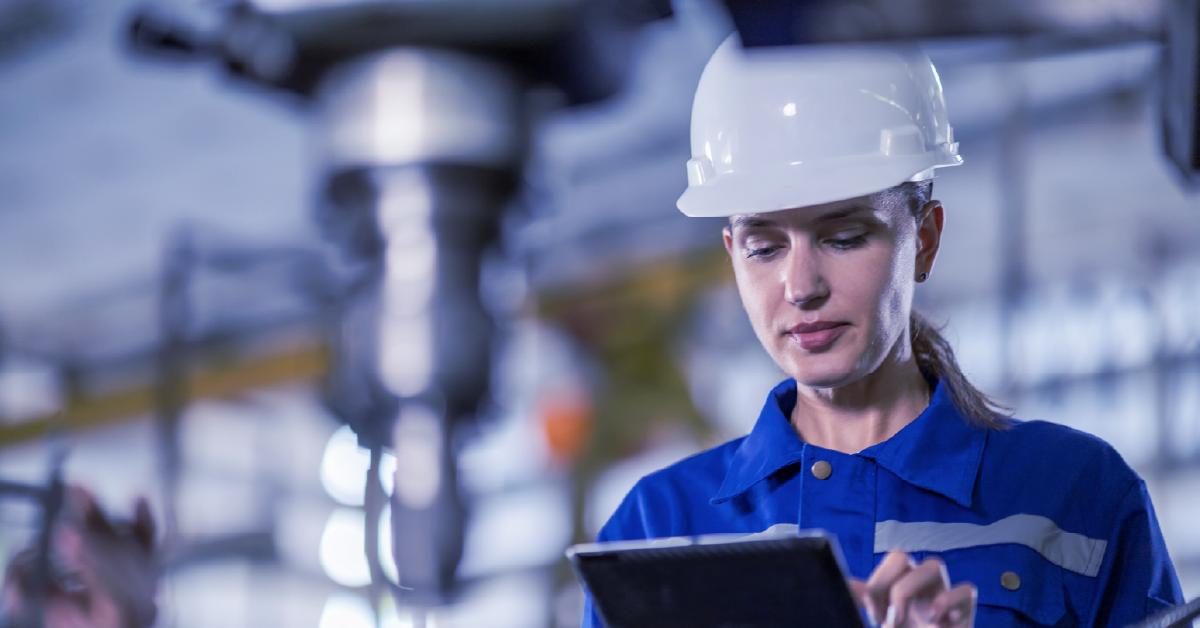
Over the past several decades, manufacturers have relied on Lean and Six Sigma initiatives to improve efficiency, quality, and overall productivity.
However, with the advent of Industry 4.0, companies are now equipped with more tools—and more data than ever—to pursue these goals. Emerging technology is generating vast troves of data, termed big data, to provide insights that are driving a paradigm shift in manufacturing today.
Of course, this opportunity brings its own challenges, which, if not effectively managed, can hamstring a company’s ability to harness the power of this data.
In this article, we explore how companies are using big data to reshape manufacturing operations, covering topics such as:
- What is big data
- How manufacturers collect big data
- The role of analytics in big data
- Applications of big data manufacturing
Download a free eBook on Cost of Quality: The Hidden Truth About Your Ultimate Quality Metric
What Is Big Data Manufacturing?
In manufacturing, big data is a term used for the massive volumes of data generated in production environments, including data produced by machine sensors, production processes, and other systems. Big data can be highly complex and variable and is often generated and delivered at high speed. Companies can harness this data for applications such as:
- Production process optimization
- Operational efficiency improvement
- Quality control
- Predictive maintenance
- Supply chain management
How Manufacturers Collect Big Data
Big data in manufacturing can be gathered at every step of the production process, such as data from equipment, devices, and even operators themselves.
Manufacturers can collect more data than ever before thanks to a combination of technologies that include:
- Industrial Internet of Things (IIoT) sensors deployed on machinery to collect data on production equipment parameters such as machine performance, environmental conditions, and energy use
- Manufacturing execution systems (MES) that capture real-time data on production processes, quality performance, and other metrics
- Enterprise resource planning (ERP) software that integrates data from multiple departments across the organization on materials, production schedules, and customer orders
- Industrial control systems like supervisory control and data acquisition (SCADA) systems and programmable logic controllers (PLCs) to monitor the production process
The Role of Analytics in Big Data Manufacturing
Big data in manufacturing is often measured by the terabyte, such that trying to make sense of the data is like trying to drink from a firehose. This simple fact puts analytics at the center of an organization’s ability to capitalize on the insights hidden in these data sets.
Again, it’s an area where emerging technology is opening up new frontiers in production optimization. Three of the most prevalent are:
- Cloud platforms: The cloud provides scalable, secure storage that allows companies to store, access, and analyze big data without capital-intensive on-premise servers.
- Advanced analytics: Tools such as statistical analysis, forecasting, and predictive modeling allow companies to aggregate and perform sophisticated assessments on historical plant floor data to optimize production processes.
- Artificial intelligence (AI): AI or machine learning systems use advanced analytics to identify relationships, patterns, and anomalies in large data sets. AI also goes further with the ability to handle unstructured data, learn from itself over time, and make autonomous decisions based on the data.
Another critical challenge in effectively leveraging big data is the ongoing shortage of data scientists. The Bureau of Labor Services cites data scientists as one of the fastest-growing careers, expected to grow 36% through 2031. Data science is a relatively new field, creating a supply chain issue regarding the expertise needed for advanced analytics or AI algorithms involving predictive analysis.
Applications of Big Data in Manufacturing
The sheer volume of information in big data combined with advances in data science has led to a proliferation of applications for big data in production environments.
Below we explore some of the ways manufacturers are using big data to support continuous improvement, including for:
- Production optimization
- Process improvement
- Tool optimization
- Increased yield
- Predictive maintenance
- Quality control
- Supply chain management
- Employee health and safety
Production Optimization
Production optimization is a critical area where organizations are looking to use big data to increase operational efficiency. In this context, historical and other data can be analyzed to:
- Improve the accuracy of demand forecasts to promote efficient production planning and resource use
- Maximize capacity while smoothing out unevenness in production schedules that causes overburden at times and underutilization at others
- Optimize inventory management to reduce costs, excess inventory, and delayed orders
Process Improvement
Big data is driving a sea change in how manufacturers monitor and adjust their processes, helping them streamline their operations to improve efficiency and reduce costs.
IIoT sensors, for example, provide a continuous stream of data plants can use for real-time monitoring of machines and manufacturing processes. This allows plants to pinpoint inefficiencies in their process, allowing them to make data-driven decisions to increase production and minimize downtime.
For instance, one automotive manufacturer was struggling with a high rate of test failures on an axle assembly line. What they didn’t know initially was that it wasn’t a production defect—it was a problem with the test circuit in one specific axle carrier. Using IIoT combined with AI, the manufacturer was able to:
- Determine based on the data which axle carrier was the problem
- Establish criteria for moving the carrier offline, such as if the carrier fails three of five tests
- Notify maintenance about the repair issue and provide a likely root cause
Tool Optimization
Normal wear and tear on tools can introduce problems in the manufacturing process, whether due to outright tool failure or simply suboptimal performance. Consider, for instance, the implications of a gauge whose measured values gradually shift over a period of time.
Proper maintenance and ongoing monitoring are crucial to ensuring tools work in the way they need to, with big data providing the ability to:
- Monitor tool parameters such as temperature, pressure, and vibration to pinpoint patterns and anomalies indicative of poor performance or impending failure
- Predict tool life based on historical and performance data to determine optimal replacement schedules that minimize unplanned downtime
- Analyze failure data to identify the root cause of failures and appropriate corrective actions
Increased Yield
Big data analytics can reveal insights that manufacturers can use to increase yield, even in organizations already performing at a high level.
One chemical manufacturer cited by McKinsey, for example, used AI to evaluate the relative impact on yield of inputs like coolant pressure, temperature, and carbon dioxide flow. Based on this analysis, the company reduced material waste by 20% and energy use by 15%, contributing to an overall increase in yield.
Predictive Maintenance
Big data combined with IIoT sensors embedded in production equipment are helping manufacturers take a more proactive approach to maintenance that reduces unplanned downtime.
For instance, IIoT sensor data can be combined with other data sources such as historical performance data, environmental conditions, and maintenance. Advanced data analytics and machine learning can then be applied to this data to:
- Identify when a piece of equipment is likely to fail
- Determine which type of maintenance is needed
- Identify the root cause of equipment failures to accelerate problem-solving
- Predict the useful lifespan of equipment
- Determine the best time to conduct maintenance based on equipment condition rather than on a fixed schedule or in reaction to problems
Quality Control
Manufacturers can leverage big data for improved quality control using various information from sensors, inspections, production data, customer feedback and more.
By effectively harnessing quality-related data, manufacturers can identify leading indicators of potential quality issues, as well as:
- Use historical data for more effective root cause analysis
- Monitor quality metrics in real-time for faster identification and correction of quality problems
- Compare relationships between different process parameters and quality performance outcomes for more effective root cause analysis and corrective action
- Develop predictive models based on historical data and performance metrics to forecast potential defects and prevent them before they occur
- Analyze supplier performance in the context of other quality metrics to predict potential issues, avoid supply chain disruptions, and improve the supplier selection process
As an example, many automotive manufacturers use layered process audits to conduct daily checks of production inputs to standardize processes and reduce defects. Manufacturers can then integrate this data with business intelligence platforms like PowerBI or Tableau to perform advanced analytics and gain predictive insights for data-driven decision-making.
Making Your Data Work for You
Digital transformation in manufacturing has created a growing number of companies rich in data but poor in insights. These organizations often have vast quantities of structured and unstructured data available to them, with limited capabilities to use it to improve their operations.
The ability to harness actionable insights using advanced analytics is quickly becoming a key differentiator among manufacturing leaders. Those overwhelmed with where to start should begin with a specific goal, gathering data on the relevant process and then applying advanced tools to solve the problem.